Congratulations to Leonie Henschel for the 1st place: Best Scientific Submission at the
2020 BVM Workshop.
Our submission Parameter Space CNN for Cortical Surface Segmentation and
YouTube presentation has convinced the reviewers.
In that work we investigate neural networks for spherical signals of the brain:
Spherical coordinate systems have become a standard for analyzing human cortical neuroimaging data.
Surface-based signals, such as curvature, folding patterns, functional activations, or estimates of
myelination define relevant cortical regions. Surface-based deep learning approaches, however, such
as spherical CNNs primarily focus on classification and cannot yet achieve satisfactory accuracy in
segmentation tasks. To perform surface-based segmentation of the human cortex, we introduce and
evaluate a 2D parameter space approach with view aggregation (p3CNN). We evaluate this network with
respect to accuracy and show that it outperforms the spherical CNN by a margin, increasing the
average Dice similarity score for cortical segmentation to above 0.9.
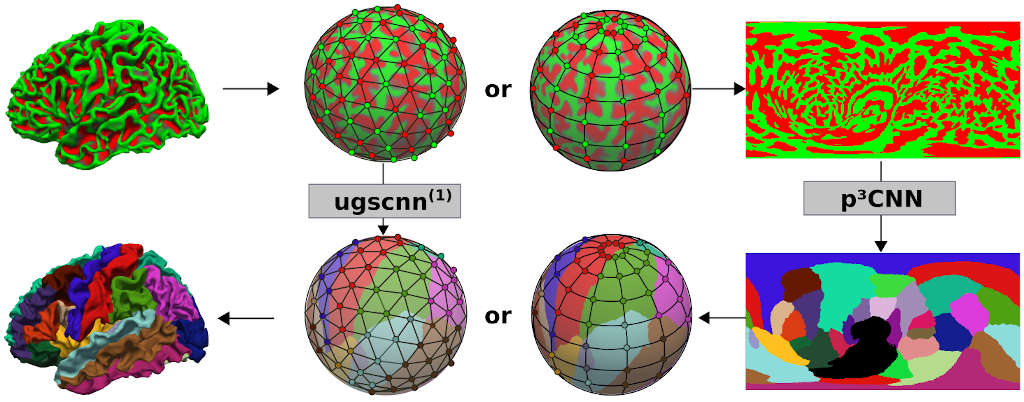
Figure: Comparison of a spherical CNN (ugscnn, middle) and our proposed view-aggregation on 2D spherical parameter
spaces (p3CNN, right) for cortical segmentation of the cortex (bottom row).
Today we finally took the time out of the always-busy schedule of writing grants and papers and created an initial version of the
DeepMI Lab Webpage. This step has long been overdue and we are happy to present it to the world.
There has been a long discussion how to solve mutliple problems at the same time:
- how can we have a webpage that is flexible, yet easy to maintain for more than one curator
- how can we represent the fact that the Lab is associated across multiple institutions
- how can we version control the page, edit quickly without local IT
- where can we host it
- and how should we even call the lab (I vetoed Reuter-Lab)
The first few points were solved by starting with github.io and jekyll - easy. The name was another beast!
The Lab’s background is in developing computational methods for medical image analysis. We did this since 2008 and recently people
like to call it AI (basically whenever a computer does something cool, like analyze an image). Most of our recent methods are
deep-learning based, extending existing architectures for specific goals, e.g. brain MRI segmentation.
This is why Deep Medical Imaging Lab (DeepMI) was selected. Yet, we are not limited to deep-learning and have a lot of work on traditional
methods (robust image registration, shape analysis, geometry processing etc). So expect to see some variety.
We hope you enjoy this site. Please contact us with any comments. We look forward hearing from you …